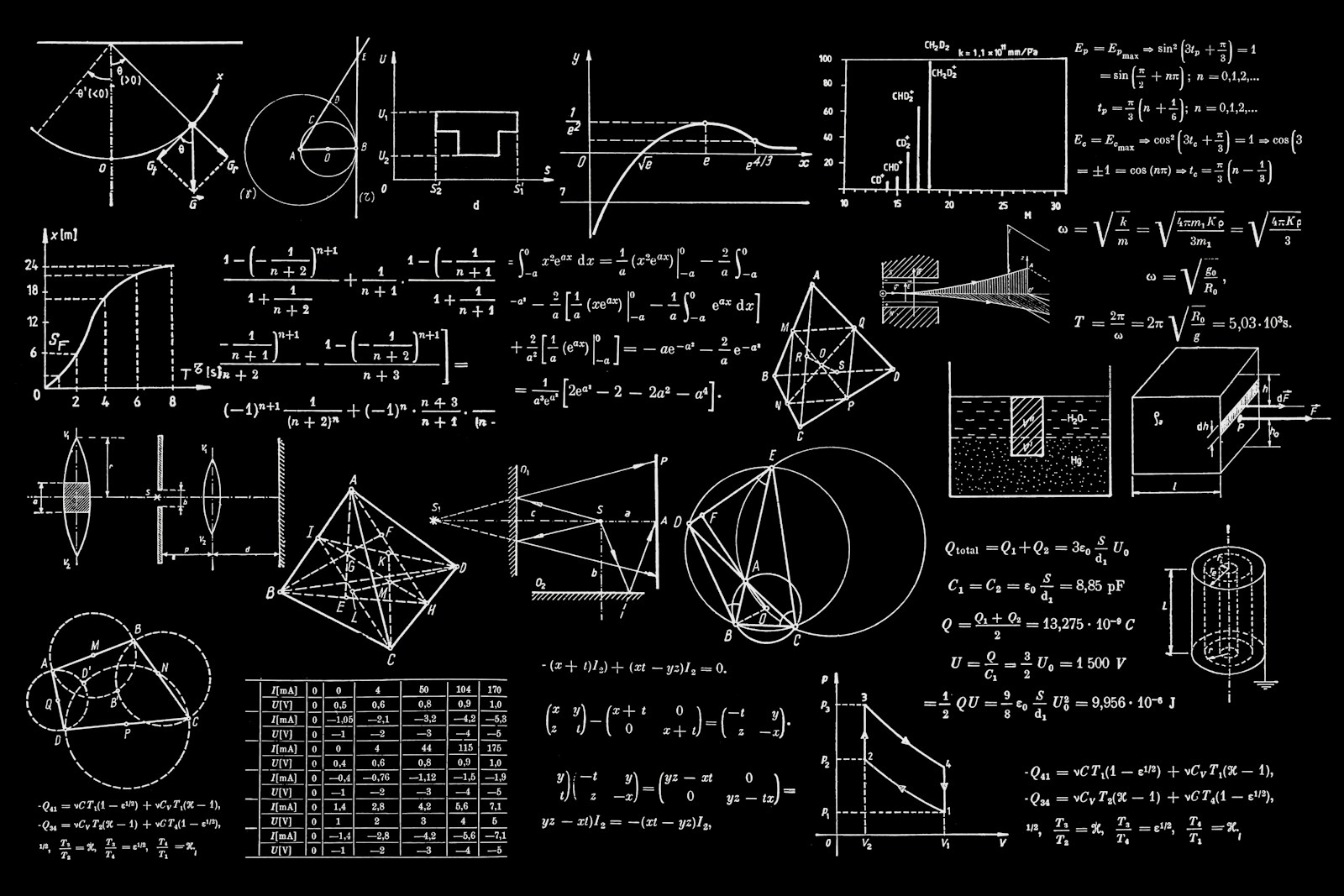
Moment Matching for Bayesian Inference in the Baseline New–Keynesian Model
HWWI Working Papers No. 4, Hamburg Institute of International Economics.
Contrary to claims in studies on financial economics, a sparse database often obscures the identification of parameters in macroeconomic models. These identification problems originate from the poorly defined mapping between a structural model and reduced-form parameters. Hence, researchers rely on prominent estimation methods, such as Bayesian approaches, which require sound knowledge of prior distributions on parameters. These approaches, however, are characterized by a flat likelihood and/or a posterior distribution driven mainly by prior information. To alleviate identification issues, we apply approximate Bayesian computation combined with the choice of specific moment conditions. This estimation approach not only allows for circumventing high dimensional likelihood functions but also avoids parameter identification problems given the use of a bootstrap method. Our estimation method is successfully applied to a hybrid version of the New Keynesian model.